The growing adoption of AI has brought with it a great deal of hype, particularly surrounding the introduction and rapid advancement of generative AI (Gen AI) capabilities.
Financial services institutions have been quick off the mark to embrace the technology in an effort to identify use cases that will accelerate business outcomes. As a result, there is a significant revenue uptick forecast: Gen AI is expected to add between $200 billion and $340 billion in value annually. That is ~4% of total industry revenue across the sector worldwide.
However, driving sustained value beyond initial pilots and proof of concepts means being able to effectively scale AI initiatives, delivering measurable outcomes and high ROI.
The question is: how?
Overcoming Implementation Challenges
An important first step involves addressing the constraints associated with the technology’s implementation – especially when it comes to integrating new platforms with legacy systems, as well as data governance and management.
Harmonizing technologies, processes, and solutions and building a robust AI core is the cornerstone of a successful AI transformation. New systems and platforms need to integrate seamlessly with existing infrastructure without causing disruptions. APIs and middleware can streamline this and bring old and new together to connect and interact smoothly.
Equally as critical as integration is powering the AI core with quality data. Data is the foundation of AI, and successful solutions and projects depend on a robust, secure data governance and management framework. A strong architecture will standardize and streamline data collection, storage, and processing, while an advanced data management platform will help ensure that data is secure, clean, accurate, and accessible to AI systems.
This is imperative to get right for fair, unbiased results. Some banks have learned this lesson the hard way.
A major US bank faced backlash in 2020 after its AI lending algorithm disproportionately rejected applications from minority communities – despite seemingly neutral data. A closer look revealed that the training data mirrored historical biases rooted in redlining and credit scoring. This highlighted the need for stringent data audits, validation, transparency, and bias mitigation through fairness testing tools.
Unlocking High-Impact Use Cases
Financial services providers have taken heed, and many are now using AI’s advancing capabilities to eliminate these types of concerns as well as to identify and implement use cases that deliver measurable results.
Key focus areas include:
1. Detecting and preventing fraud and financial crime.
Scams and fraud are on the rise worldwide and becoming increasingly sophisticated. The banking industry has proven to be one of the most vulnerable. The size of extreme losses that banking and financial services organizations have experienced has more than quadrupled since 2017, rising to $2.5 billion.
The consequences of these incidents have a ripple effect, threatening consumer confidence and impacting financial and economic stability at a wider level. That’s why financial institutions are investing in specific use cases to address the challenge.
A global financial leader uses an AI system, DocLLM, to analyze vast amounts of data in legal documents and identify and prevent potential fraud by detecting anomalies and red flags.
Another harnesses natural language processing (NLP) models to analyze transaction criteria such as amount, currency, and destination and then report anomalies and suspicious transactions. Machine learning helps train the model to classify transactions correctly, and the system has had various successes, including uncovering a major case of organized crime, money laundering, and tax evasion.
A multinational payment card service company uses a Gen AI-based platform to examine how cardholders spend money, determine the probability of fraud for every transaction as it happens, and then stop suspicious transactions before they’re processed. The platform has already helped the business score and approve over 143 billion transactions per year.
2. Augmenting investment decision-making and management.
In today’s environment where most financial institutions are challenged for profitability, generating positive investment returns is even more critical..
Advancing AI-driven NLP capabilities and large language models (LLMs) can help enhance decision-making and management of investments by processing and analyzing complex financial data rapidly and accurately. These capabilities can help spot patterns and trends that then inform and facilitate the optimization of investments.
A case in point: Two University of Florida finance professors trained LLMs to analyze both positive and negative stock price movements using news headlines and predict returns. Results showed that AI systems are capable of sophisticated return forecasting and have the power to improve financial and wealth advisory through predictive analytics.
3. Improving regulatory compliance and operational efficiencies.
Navigating the regulatory landscape is complex, time-consuming, and traditionally requires extensive manual effort.
AI can help process vast amounts of data, generate reports, and monitor transactions and operations – helping with compliance to both local and global regulations and simultaneously driving efficiencies.
Sutherland has collaborated extensively with industry players in this space.
We partnered with a leading private label credit card (PLCC) issuer in the card industry. Backed by our deep understanding of the card industry’s operating model, we developed an end-to-end solution using hyperautomation to ensure the client could seamlessly handle back-office transactions with a focus on improving compliance.
Underpinning our approach were two of our proprietary platforms: Sutherland Robility® and Sutherland Extract, which utilized AI-enabled data extraction, RPA bots, workflows, and a command center to monitor virtual workforces 24/7.
Since implementing the solution, we’ve processed 80% of the company’s back-office transactions, accelerated operational efficiency, and improved compliance and customer satisfaction, enabling the client to manage much larger volumes at a fraction of the cost.
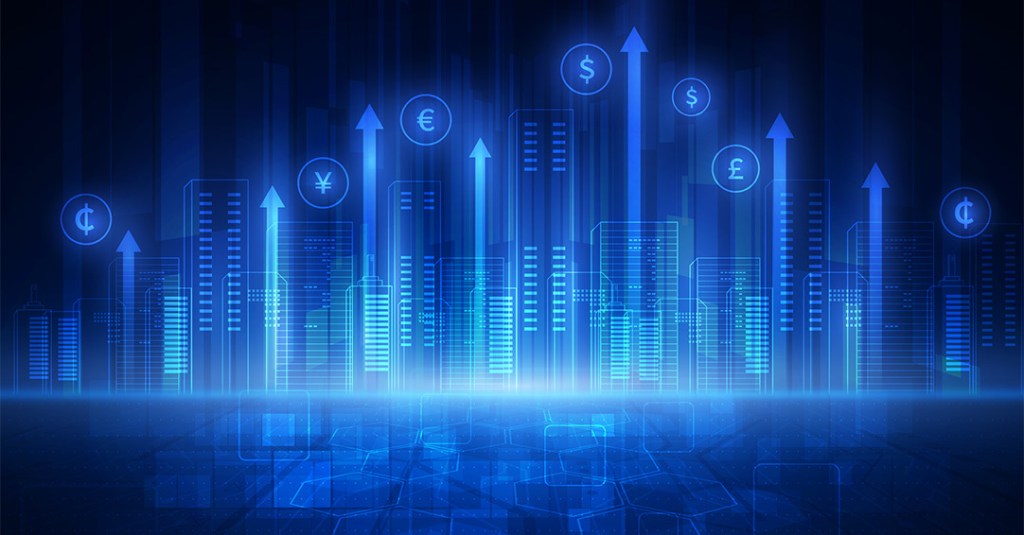
Considerations To Get from POC to ROI
To get to the point where banks can truly unlock this type of measurable ROI from their AI use cases and investments, there are both short-term and long-term considerations they will need to prioritize.
In the short term, financial institutions should:
- Look for low-hanging fruit and quick wins. Many banks start their AI journeys by automating repetitive processes. For example, using AI-powered chatbots to handle customer experience (CX) requests or automating Know Your Customer (KYC) processes. These solutions offer immediate value by reducing manual effort and enhancing efficiency.
- Focus on content synthesis and content generation. AI tools can help financial institutions create, optimize, and curate various types of content. The ability to analyze user data at speed can also drive understanding of behavior and preferences, enabling dynamic, tailored messaging, product offers, and recommendations that elevate the customer experience.
- Run pilot projects with clear KPIs. Testing AI and automation solutions in a controlled environment will showcase how the solution performs in a real-world setting, which allows banks to measure impact, identify and resolve issues, and refine the approach according to the targets set.
In the long term, banking and financial services institutions should:
- Invest in workforce AI training. Employees across the organization need to understand the impact of AI on their roles and get trained to work with the technology through AI literacy, upskilling, and reskilling initiatives. This will help integrate AI into daily operations and foster an innovation-friendly environment.
- Conduct client behavior analysis, hyperpersonalization, and risk management. Data-driven insights around customer behavior, preferences, interests, and risk profiles can help banks drive pricing optimization, introduce innovative tailored products, and improve business strategies for better outcomes.
- Embed AI in operating models. Enhanced use of AI in core processes will improve speed and agility, accelerate innovation in portfolio management strategies, open new revenue streams, and allow financial institutions to capture market share.
The Inside Track to Realizing the Value of AI
Even though banks and financial services organizations have largely embraced the adoption of AI to drive operational efficiencies and improve customer-facing functions, that investment hasn’t yet yielded its full return.
Banks need to act today to gain a strategic edge. Increasingly, that means not doing all the heavy lifting yourselves and trying to build the necessary capabilities and skills in-house. Collaborating with a partner with the core expertise to create digital chemistry that delivers measurable results can help you deliver real ROI on your AI investment exponentially faster.
Move Beyond the Hype and Unlock Transformative Banking Outcomes With AI
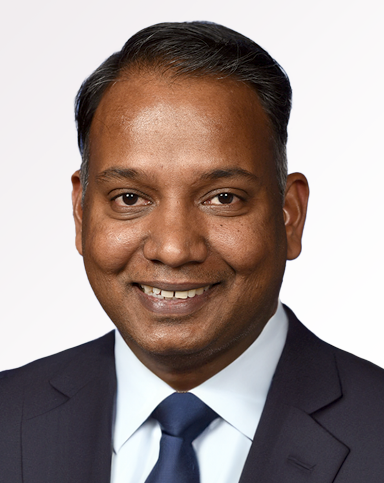
Banwari Agarwal is the CEO of Banking, Insurance, Retail, Manufacturing, Travel, and Logistics at Sutherland. Banwari brings deep expertise in digital technologies and operations and over 25 years of leadership experience across the US, Europe, and APAC. His strategic vision has driven transformative outcomes in digital business services across multiple industries, delivering innovative, cutting-edge solutions in finance, HR, procurement, and supply chain management.