For far too long, making the most of customer care investments has meant optimizing for traditional metrics like customer satisfaction (CSAT), average hold time (AHT), net promoter score (NPS), and first call response rates (FCR).
While this has taken our industry a long way — the experiences of countless millions of customers have improved greatly as a result — its effectiveness has stalled. And we’re all looking to ‘what’s next’ to drive future gains.
The good news? We don’t have to look far. The future has already arrived in the form of ‘AI-driven everything’…and that includes customer analytics.
Order-of-magnitude advances in technology, and unrelenting calls to turn contact centers into revenue generators from operational burdens, are making it possible to entirely reimagine the way we measure, monitor, and direct customer care outcomes.
Recent advances in the effectiveness and use cases of artificial intelligence (AI) are both pointing out the limitations of traditional customer care metrics and making it possible to dream up whole new sets of measurements to take their place.
It’s Time to Rethink CX Metrics As We’ve Known Them
Traditional CX metrics are often limited by their accuracy and usefulness. You know. You’re living it. Some cases in point:
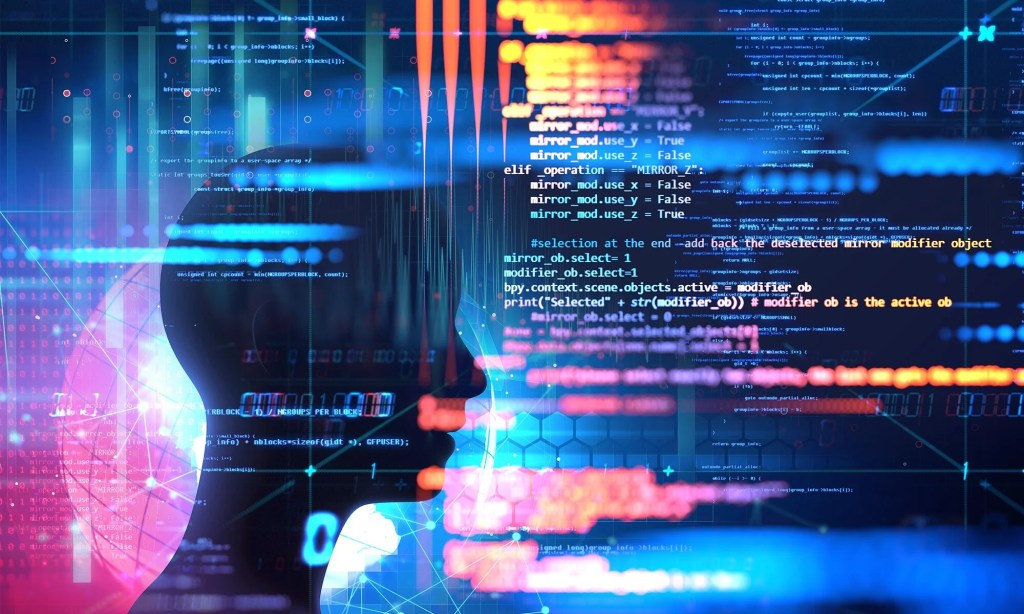
Low average handle time scores can’t be taken at face value.
A customer care agent at a software company, for example, may exceed the industry’s AHT by taking extra time to convert a customer complaint into a sale. Most likely the resultant revenue would outweigh any savings that might have resulted from a shorter call focused solely on problem resolution. But how do you capture that value-add in the AHT metrics?
First-call resolution rates tell us very little.
Just because a customer didn’t follow up on his initial contact about an issue doesn’t mean he was happy with the outcome. All that might have been resolved was that a “final sale, no refund” purchase of a wireless router was indeed nonrefundable. Meanwhile, the router’s complicated setup procedure, which was the reason the customer wanted to return the item, remained complicated, even though the agent successfully walked the customer through the installation steps. Ultimately, the call left the customer frustrated, due to the lengthy setup explanation. But because the customer didn’t need to call back, the interaction resulted in a misleadingly positive FCR score.
Net promoter scores are opaque if you can’t see what informs them.
NPS is of equally limited value when you consider that 52 percent of all people who actively discourage others from using a brand also actively recommend it.[1] Plus, the score itself offers no actionable insights about why the customer would recommend a brand. Nor does it reflect demographic factors. Perhaps the same customer would recommend a particular fantasy sports game to a peer, but not to his adolescent son.
Survey-based satisfaction scores provide a limited window into what customers truly think.
Unlike post-interaction surveys, with their highly limited questions, AI-powered sentiment analysis will capture and reflect the full range of dynamics affecting a customer’s satisfaction or dissatisfaction on a call. It will also detect and decode contextual information and unarticulated emotional cues from the customer. Just as importantly, all of this information can be relayed to the agent while the interaction remains fresh in that person’s mind. This is in sharp contrast to most CSAT surveys, which usually take several days to get converted into agent feedback. By then, the optimal teachable moment has passed. So has the chance to steer customer interaction in a revenue-generating direction.
Applying a Digital-First, AI-Powered Approach to Exceptional Results
Increasingly, brands are applying AI-powered analytics on 360-degree customer interaction data. Along the way, they’re coming up with altogether new customer measures – and measures of success.
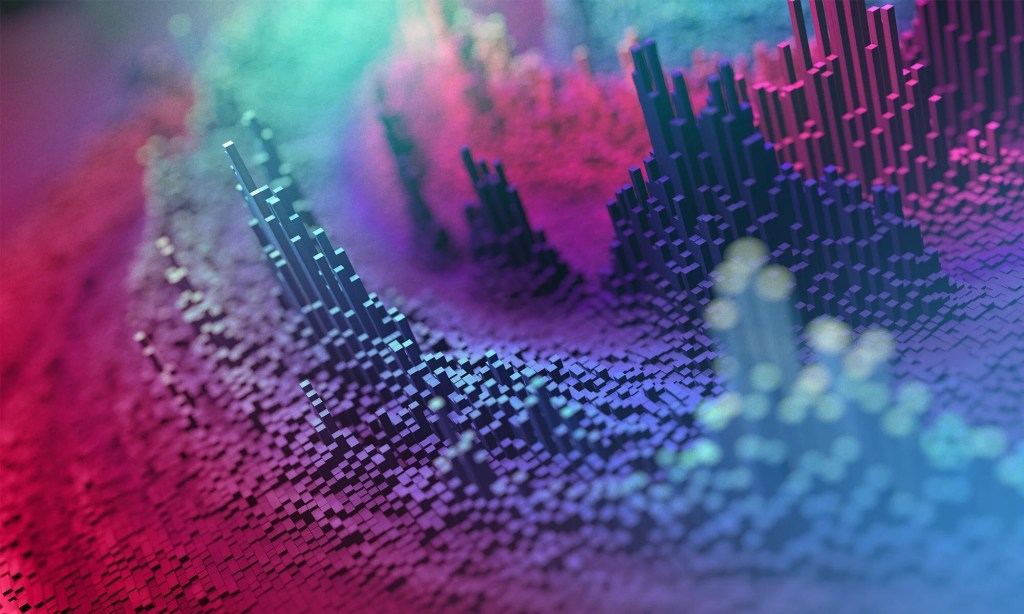
Organizations are moving from siloed to integrated 360-degree customer data. These include omnichannel interaction data across voice, chat, messaging, email, social channels, etc. To gain customer journey insights at the interaction level, it is important to not just analyze the metadata around the interaction but the actual unstructured speech and text data as well.
And they’re moving from gaining descriptive intelligence on as little as 3% of their interactions to predictive intelligence on a full 100% of those interactions. With rapid advancements in AML and NLP science, along with big data cloud-based technologies, it is possible to uncover actional strategic and operational insights from the intricacies of each customer interaction, be it at your contact center or across your digital assets on a mobile app or the web.
Here’s how they are doing it.
Mining 360-Customer Interaction Data
Leading brands are pioneering a better approach to CX — one that takes full advantage of the wealth of data now available. 360-degree customer interaction data is just not the voice/chat data at your contact center but is a combination of all the interactions that a customer has at every enterprise touchpoint. This could include stored data from feedback systems, surveys, social media, focus group research responses, transactional data, and much more.
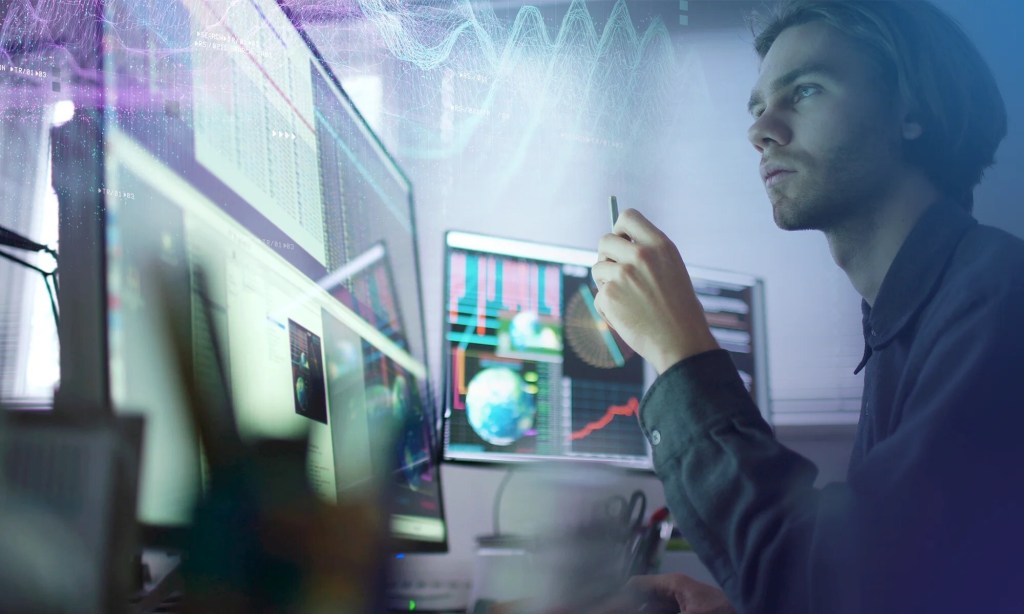
Comprehensive and dynamic customer-level data sets allow the organization to map and track customer behavior across interactions, transactions, and operations — supporting efficient real-time reporting so you can take the right corrective action at the right time.
Discovering Predictive Intelligence
Organizations with an eye to the future are boosting their AI-powered data and analytics capabilities — harnessing predictive insights to anticipate customer needs and generating the next best actions for agents based on customer information. This allows your organization to transform its customer service with outcome-based metrics like predictive CSAT, predictive FCR, and predictive sales — metrics that can differentiate both your brand and business performance.
Actioning Real-Time Conversation Insights
Today’s contact center AI can analyze customer conversations in real-time — filtering for key moments and trends to enable rapid coaching by managers. Other insights can involve text mining, key moment detection, and turn-by-turn predictive NPS.
Capitalizing on Customer Journey Insights
Using customer experience data and mapping it back to specific touchpoints is how you start to understand the key moments that influence customer behavior. With a sophisticated customer journey insights platform, you can pinpoint issues in real-time. You can test new approaches and see their impact upon customer experience (and your bottom line), using insights that update as quickly as you need them.
Analytics Success Gets Funded (Even In a Downturn)
Programs that don’t help drive sales and profitable growth typically get cut when the economy slows. This is why customer care leaders must be able to explicitly connect customer-focused projects to financial success to keep (or grow) funding and attract support for new initiatives.
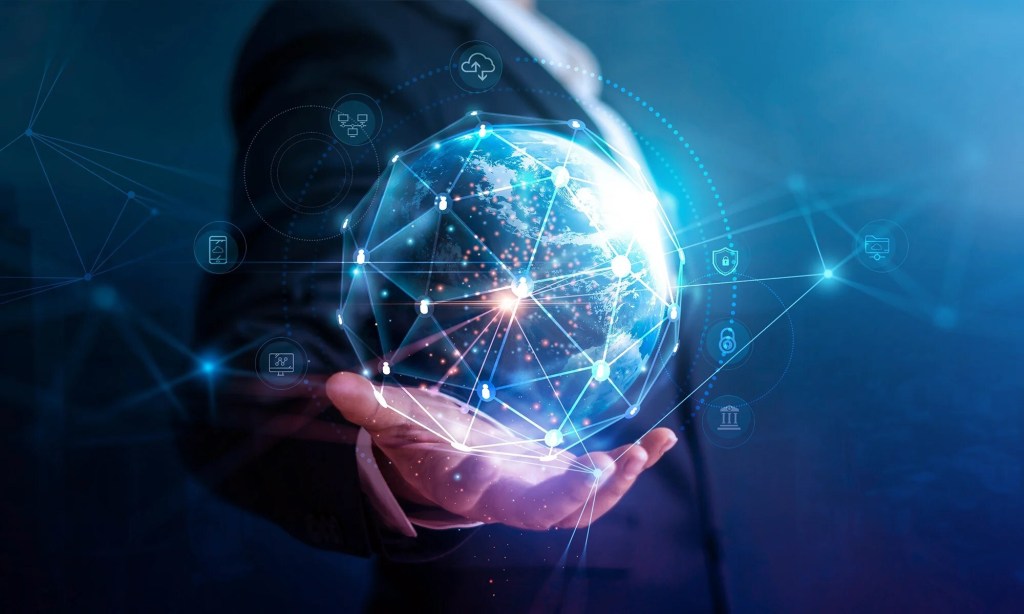
Investing in data analytics to power CX-drive growth is investing in the right priorities. And organizations that are optimizing their AI-driven customer analytics efforts are seeing some remarkable results.
To learn more about how the future of customer care will see new CX horizons as a result of AI-powered analytics applied to 360-degree customer interaction data, check out this article. Or see our whitepaper, Customer Care in 2030: Where the Future is Heading.
A good place to start making all the ideas a reality is a simple use case with well-defined success measures and outcomes. The process of evaluating, selecting, and putting technology to the test through a pilot or proof of concept empowers teams to build a blueprint for working together. It also fosters a builder mindset within the business.
Let’s talk. We’d love to hear from you.
1 https://hbr.org/2019/10/where-net-promoter-score-goes-wrong